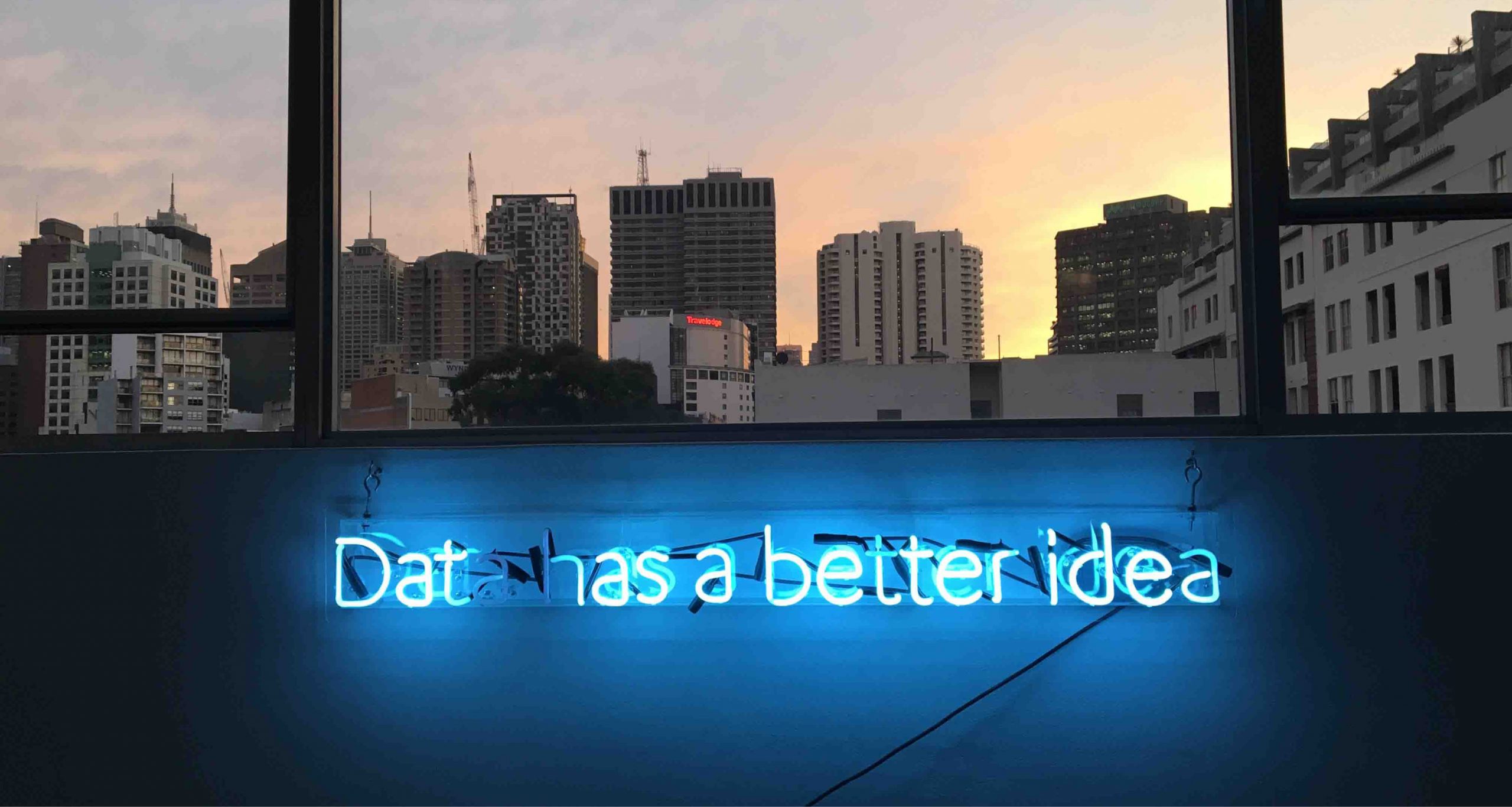
Investment Management in the Age of Artificial Intelligence
By: Defred Folts III, Managing Partner, Chief Investment Strategist
June 2018
Artificial Intelligence and machine learning are everywhere. In the words of Tad Friend at The New Yorker magazine, “AI has grown so ubiquitous -- owing to advances in chip design, processing power and big-data hosting that we rarely notice it.” Creating AI tools to solve problems goes back to the work of the mathematical genius Alan Turing in the 1940’s. Turing was the character featured in the movie “The Imitation Game,” in which he and fellow mathematicians attempt to break the Nazi’s Enigma code during World War II. Today, there are many different flavors of AI including; ANI—artificial narrow intelligence, AGI—artificial general intelligence, and the current holy grail, AI--deep learning.
AI and machine learning are also hot topics in the investment management industry with constant references in the financial press to man vs. machine, human vs. digital, and how AI and machine learning are about to transform the way that we invest. There is little doubt that the investment industry will be transformed by this technology. However, at 3EDGE Asset Management, we believe that the argument over human vs. machine is a false choice, and that the best way forward is human plus machine. We view the relationship between human and machine intelligence as one of cooperation rather than competition.
As the man in the TV commercial says, at 3EDGE, “we know a thing or two, because we’ve seen a thing or two,” about applying Artificial Intelligence and machine learning to investment management. In fact, it is in many ways foundational to our investment approach. However, we also believe that when applying AI and machine learning tools to investment management, it is critical to understand that these tools can be very good at certain tasks, while they may not be so good at others. In particular, AI and machine learning tools are quite good at analyzing massive amounts of data and detecting patterns. However, they are often not yet good at being able to differentiate between correlation and causation, and in investment management that can be particularly problematic.
There are many industries where AI and machine learning are already dependable and powerful analytical tools, such as in the field of consumer marketing -- think Facebook, Amazon and Alphabet (Google). However, in more nonlinear, complex environments such as the eco-system that is the global capital markets, black box machine learning tools can prove to be unreliable. Too often traditional, quantitative black box investment models are almost entirely data driven and thereby inductive in nature. In these types of models, large amounts of historical data are collected and analyzed using regression-based modeling techniques and then the model output is evaluated using back-tested results. This approach can be fraught with risks and is oftentimes unreliable for analyzing the behavior of a complex, nonlinear system like the global markets.
“For years the dream of many researchers was to create an artificial intelligence that could rival that of people. However, we’re seeing that AI is instead becoming a tool to extend our own human capabilities. Never before in history have our tools been so responsive to us, and we to our tools.”
“Human + Machine – Reimagining Work in the Age of AI”,
R. Daugherty and H.J. Wilson,
Harvard Business Review Press, 2018
What exactly is Artificial Intelligence and machine learning?
To better understand AI and machine learning I decided to seek out someone who has been working in this area for decades, and so I paid a visit to Bob Phillips, Managing Partner, Chief Technology Officer and member of the investment committee right here at 3EDGE Asset Management. Bob is responsible for all of the 3EDGE investment modeling platforms. He holds several patents and has over 30 years of experience in applying Artificial Intelligence and machine learning tools to solving complex problems. Bob began with a working definition: “Artificial Intelligence (AI) can be defined as the ability of a digital computer or computer-controlled robot to perform tasks commonly associated with intelligent beings.” From there he went on to explain more about AI and machine learning in general, and about how we utilize the power of Artificial Intelligence and machine learning at 3EDGE. To date, work on AI has focused on two different approaches, expert systems and machine learning. Expert systems seek to capture the rules humans use to solve specific problems and these tools have already been successfully applied in a number of domains including medical diagnostics. Expert systems also have the ability to explain the resulting conclusions by tracing back through the logical framework, thereby enabling the model’s human collaborators to intuitively understand why the model’s output is what it is. However, this approach may be limited in terms of scale by the challenge of assembling a comprehensive rule set even in limited domains. This means that, as problems grow larger and more complex, with a larger set of inputs, the ability for any intuitive understanding of the model grows increasingly more challenging.
Machine learning, on the other hand, attempts to directly model the neural connectivity of the human brain. The underlying algorithms are independent of the specific problem domain and must be trained using extensive data sets from the domain. With extensive training these models can provide precise predictions if they are able to identify a matching pattern. This approach is commonly used today for visual recognition and classification, product recommendations and game playing. However, unlike the expert systems approach, machine learning models cannot explain the logic behind the results.
How we use AI and machine learning at 3EDGE
Our investment approach combines aspects of both traditional expert systems and machine learning. We utilize Artificial Intelligence tools and techniques to leverage the combination of causal relationships and behavioral logic in our model. We begin by identifying causal expressions derived from a logical framework for how value is created or destroyed in the global capital markets. This logical framework is built using principals of system dynamics to understand the forces that drive the flow of capital through the global markets as a collection of nonlinear differential equations where each force component is derived from market-based data. This logical framework produces forward-looking risk-adjusted projections for each asset class that we model. In addition to the casual relationships, we also capture a linked set of classical expert system style, conditional rules that define behavioral market characteristics.
The next critical step is to determine the relative weights for each of the causal forces and the parameters and thresholds associated with the behavioral characteristics. Since our ultimate task is to construct portfolios within our targeted ranges for expected risk-adjusted return characteristics, we use a machine learning approach to establish the weights, parameters and thresholds. This is done by applying the model described above to every month starting in 1871 up to the present. For each time period portfolios are constructed, and three characteristics are calculated, CAGR (compound annual growth rate), Sharpe Ratio and Maximum Drawdown for each portfolio by evaluating the portfolio against the known returns. The three characteristics are combined into a multiple objective function. For risk management purposes, there is greater emphasis on the maximum drawdown component of these calculations relative to the weights of the other two characteristics. This is then used as the metric for model optimization. Importantly, this process should not be confused with traditional portfolio optimization.
The model optimization process uses machine learning algorithms combining Genetic and Steepest Descent to search the parameter space. One way to think about a genetic algorithm is that it is somewhat similar to the way that we observe an evolutionary process, while a steepest descent algorithm looks for the nearest and most optimal path upwards in fitness based on its current location.The resulting model has the advantage of using a very large training dataset for precision and the explain-ability of an expert system since at its core ours is a causal model.
Caveat Emptor
As we explained earlier in this commentary, in the world of investment management, AI and machine learning tools come mostly in the form of a quantitative black box, wherein the model is entirely data- driven and it isn’t possible to explain the logic behind the results. In our estimation, this is one of the reasons that machine learning tools applied to investment management can be problematic. Understanding the reasons behind the predictions from an AI based model is critical in order to be able to trust the output and feel comfortable in making actual investment decisions based upon the model output. In the nonlinear, complex world of the global capital markets understanding why models reach the conclusions they do is critical.
AI and neural networks are designed to learn like the human brain, but one has to be careful. It is critical to make sure that these models learn correctly.This is why the 3EDGE approach has been to combine deductive (real human) with inductive (machine) intelligence. In fact, in many ways the most difficult and time-consuming work is to first develop the theory behind the true cause and effect relationships that drive the global capital markets--deductive and only then to employ AI and machine learning tools to test those theories over vast time periods. It is important to know that Steve Cucchiaro, President and CIO of 3EDGE Asset Management, along with Bob Phillips and other members of the 3EDGE research and investment team have spent decades studying and seeking to understand the true cause and effect relationships that drive the global capital markets.
Example of an AI tool learning incorrectly
One example of how machine learning could be dangerous if not used properly involves the development of a deep learning, neural network that appeared to learn to differentiate between dogs and wolves. Since such a feat involves recognizing fairly subtle differences between the two it seemed to be a rather impressive feat. However, what the folks who did this work eventually discovered was that the neural network wasn’t actually learning the physical differences between dogs and wolves. Instead, what the model actually learned was that the vast majority of the pictures of wolves were taken on snow while the pictures of dogs were taken on grass. The neural network had learned to differentiate between the two species of animals by differentiating between the snow and the grass in the background of the photos. Obviously, the network had learned something, but it had learned incorrectly. If the dog had been on snow or the wolf had been on grass, it would have gotten the wrong answer. This example highlights how a lack of explain-ability in this deep learning, neural network led to problems with the results of the model. It illustrates the importance ofmaintaining transparency through to the how and the why behind the model’s decision-making process.In this particular case it was possible to ultimately recognize and correct the error in the model, but what other errors haven’t yet been uncovered?
Summary
There can be no doubt that Artificial Intelligence and machine learning are powerful tools that will change the world we live in, well beyond what we can presently imagine. At 3EDGE we utilize the power of both Artificial Intelligence and machine learning as foundational constructs of our investment research and portfolio management process. However, we also believe that in the eco-system that is the global capital markets, the concept of human vs. machine is a false choice and that it is the combination of human plus machine, deductive plus inductive, that provides 3EDGE with a competitive advantage over the more common black box quantitative models that are now so prevalent in the investment world.
DISCLOSURE: The opinions expressed in this commentary are those of DeFred G. Folts III, Chief Investment Strategist of 3EDGE Asset Management, LP, and are subject to change without notice in reaction to shifting market conditions. The opinions are not intended to provide personal investment advice and do not consider the investment objectives and financial resources of the reader. These observations include information from sources 3EDGE believes to be reliable, but the accuracy of such information cannot be guaranteed. Investments in securities, including common stocks, bonds, commodities and ETFs, involve the risk of loss that investors should be prepared to bear. Past performance may not be indicative of future results.